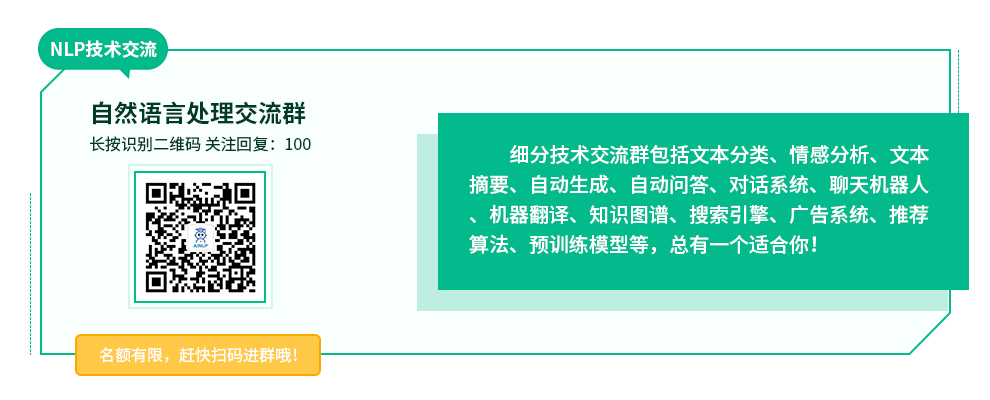
自然语言处理:单词计数
Natural Language Processing: (Simple) Word Counting
作者:Regina Barzilay(MIT,EECS Department, November 15, 2004)
译者:我爱自然语言处理(www.52nlp.cn ,2009年1月10日)
三、 语料库相关
a) 数据稀疏问题(Sparsity)
i. “kick”在一百万单词中出现的次数(How often does “kick” occur in 1M words)?——58
ii. “kick a ball”在一百万单词中出现的次数(How often does kick “kick a ball” occur in 1M words)?——0
iii. “kick”在web中出现了多少(How often does “kick” occur in the web)?——6M
iv. “kick a ball”在web中出现了多少(How often does “kick a ball” occur in the web)?——8.000
v. 数据永远不会嫌多(There is no data like more data)
b) 非常非常大的数据(Very Very Large Data)
i. Brill&Banko 2001:在混合集合消歧任务中通过增加数据规模的方法进行训练所得到的结果比在标准训练语料上训练的最好系统的结果好很多(In the task of confusion set disambiguation increase of data size yield significant improvement over the best performing system trained on the standard training corpus size set)
1. 任务(Task):对“too,to”这样的词对进行歧义消除(disambiguate between pairs such as too, to)
2. 训练规模(Training Size):从一百万词到10亿词不等(varies from one million to one billion)
3. 用于对比的学习算法(Learning methods used for comparison):winnow算法,感知器算法,决策树算法( winnow, perceptron, decision-tree)
ii. Lapata&Keller 2002, 2003:web可用做非常非常大的语料库(the web can be used as a very very large corpus)
1. 计数可能被噪音干扰,但是对于一些任务这不是什么大问题(The counts can be noisy, but for some tasks this is not an issue)
c) 布朗语料库(The Brown Corpus)
i. 著名的早期语料库(Famous early corpus) (Made by Nelson Francis and Henry Kucera at Brown University in the 1960s)
1. 一个关于美国书面语的平衡语料库(A balanced corpus of written American English),包括报纸,小说,非小说,学术等体裁(Newspaper, novels, non-fiction, academic)
2. 一百万单词数,500份文本(1 million words, 500 written texts)
3. 你认为这是一个大型语料库吗(Do you think this is a large corpus)?
ii. 注,关于布朗语料库更详细的介绍:
1. 20世纪60年代,Francis和Kucera在美国Brown大学建立了世界上第一个根据系统性原则采集样本的标准语料库——布朗语料库。
2. 主要目的是研究当代美国英语
3. 按共时原则采集文本的语料库,只选录1961年间由美国人撰写出版的普通语体的文本。
4. 规模为100万词次,全部语料分成15种体裁,共500个样本,每个样本不少于2000词次。
5. TAGGIT系统:词类标记81种,正确率达77%
6. 语料分A-R共18种类型,A-J属于资讯类语体,K-R属于想象类语体
例:A 报刊:新闻报道;B 报刊:社论…
7. 样本通过随机采样方法得到。首先从各类体裁目录中按样本数要求随机选出进入语料库的文本,然后从选出的文本中随机截取不少于2000词次的片断作为样本,采样时要保证最后一个句子是完整的
8. 版本:A,B,C,卑尔根I,卑尔根II,布朗MARC
9. 布朗语料库从语料库的整体规模,语料的分布和语料的采样上都经过了精心的设计,一致被公认为是一个能反映语言共性的平衡语料库。
d) 近年来的语料库(Recent Corpora)
语料库(Corpus) 规模(Size) 领域(Domain) 语言(Language)
NA News Corpus 600 million newswire American English
British National Corpus 100 million balanced British English
EU proceedings 20 million legal 10 language pairs
Penn Treebank 2 million newswire American English
Broadcast News spoken 7 languages
SwitchBoard 2.4 million spoken American English
ii. 了解更多语料库的信息,请查询语言数据联盟(For more corpora, check the Linguistic Data Consortium):
http://www.ldc.upenn.edu/
e) 语料库内容(Corpus Content)
i. 类型(Genre):
– 新闻,小说,广播,会话(newswires, novels, broadcast, spontaneous conversations)
ii. 媒介(Media):文本,音频,视频(text, audio, video)
iii. 标注(Annotations):tokenization, 句法树(syntactic trees), 语义(semantic senses), 翻译(translations)
f) 标注例子(Example of Annotations): 词性标注(POS Tagging)
i. 词性标注集对简单的语法功能编码(POS tags encode simple grammatical functions)
ii. 几个词性标注集(Several tag sets):
1. Penn tag set (45 tags)
2. Brown tag set (87 tags)
3. CLAWS2 tag set (132 tags)
iii. 举例:
Category Example Claws c5 Brown Penn
Adverb often, badly AJ0 JJ JJ
Noun singular table, rose NN1 NN NN
Noun plural tables, roses NN2 NN NN
Noun proper singular Boston, Leslie NP0 NP NNP
g) 标注中的问题(Issues in Annotations)
i. 同样的认为不同的标注方案很正常(Different annotation schemes for the same task are common)
ii. 在某些情况下,方案之间有直接的映射关系;在其他情况下,它们并没有显示出任何关系(In some cases, there is a direct mapping between schemes; in other cases, they do not exhibit any regular relation)
iii. 标注的选择是由语言,计算和/或任务需要驱动的(Choice of annotation is motivated by the linguistic, the computational and/or the task requirements)
未完待续:第四部分
附:课程及课件pdf下载MIT英文网页地址:
http://people.csail.mit.edu/regina/6881/
注:本文遵照麻省理工学院开放式课程创作共享规范翻译发布,转载请注明出处“我爱自然语言处理”:www.52nlp.cn
本文链接地址:
https://www.52nlp.cn/mit-nlp-second-lesson-word-counting-third-part/
There is no data like more data似应翻译为“数据永远不会嫌多”
[回复]
admin 回复:
10 4 月, 2009 at 12:02
非常感谢,已经在文章中更新了!
[回复]